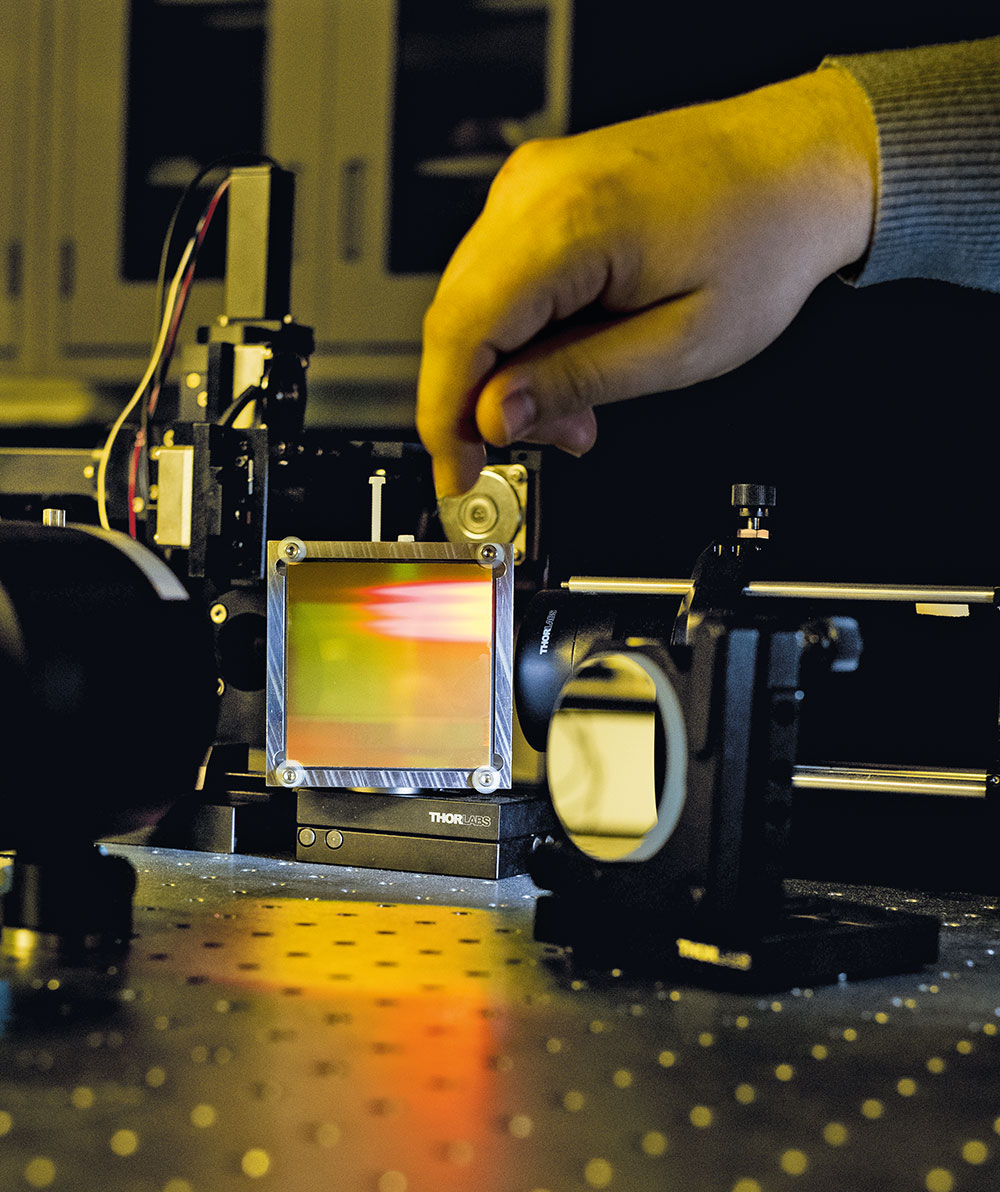
Feature
The tools that drive discovery
What do scientists do when their most pressing question cannot be answered with existing technology? Make their own from scratch, of course.
By Joshua A. KrischJeffrey Demas adjusted his protective eyewear and studied the bulky vertical band saw. He was about to shape a sheet of aluminum into a bracket—the potential solution to a problem he had been wrestling with for years.
A postdoc in the laboratory of Alipasha Vaziri, Demas was perfecting a new microscope that would make it possible to watch brain processes unfold with unprecedented speed and precision. The technique relied on a scanner that enabled researchers to capture crisp images at different depths in brain tissue and detect thousands of neurons firing at once. But as Demas had learned the hard way, the scanner had to be precisely aligned—so precisely, in fact, that even a few hundred microns off-kilter would turn precious data into meaningless noise. To keep the precarious scanner in place, he needed a bracket with the right dimensions. And it did not exist.
In the Precision Instrumentation Technologies (PIT) resource center at The Rockefeller University, under the watchful eye of the center’s instrumentation engineer, James Petrillo, Demas made a few final adjustments to the band saw. “Let’s give it a shot,” said Petrillo, raising his voice over the thrum of the saw whirring to life. “Hopefully we don’t–”
A buzz, a crash. Silence. “Not your fault,” Petrillo laughed, raising his safety goggles to survey the damage. “It’s a temperamental machine.” With a shrug, Demas retired to a nearby desk, reconsidering with pen and paper how he might manufacture his elusive bracket.
Biologists need highly sophisticated tools to answer their most burning questions—and more often than not, those tools cannot be found on any vendor website. This means researchers must venture on elaborate detours, working with engineers—or moonlighting as engineers themselves—to create instruments that fit their needs. Sometimes a new device can be fashioned with a band saw; in other cases, solving problems at the cutting edge requires a less conventional tool—a novel biochemistry approach or advanced computer software, for example. And fashioning a new instrument or technique is almost never straightforward.
“Only a handful of scientists are committed enough to develop new tools when they hit a barrier in their research,” says Nathaniel Heintz, the James and Marilyn Simons Professor, a neuroscientist and innovator. “But if they do it well, it pays off. For a period of time—before their technology is replaced with a better one—these people are allowed to see things that others have never been able to see before.”
Here are four tools in the bioscience vanguard.
The no-compromise microscope
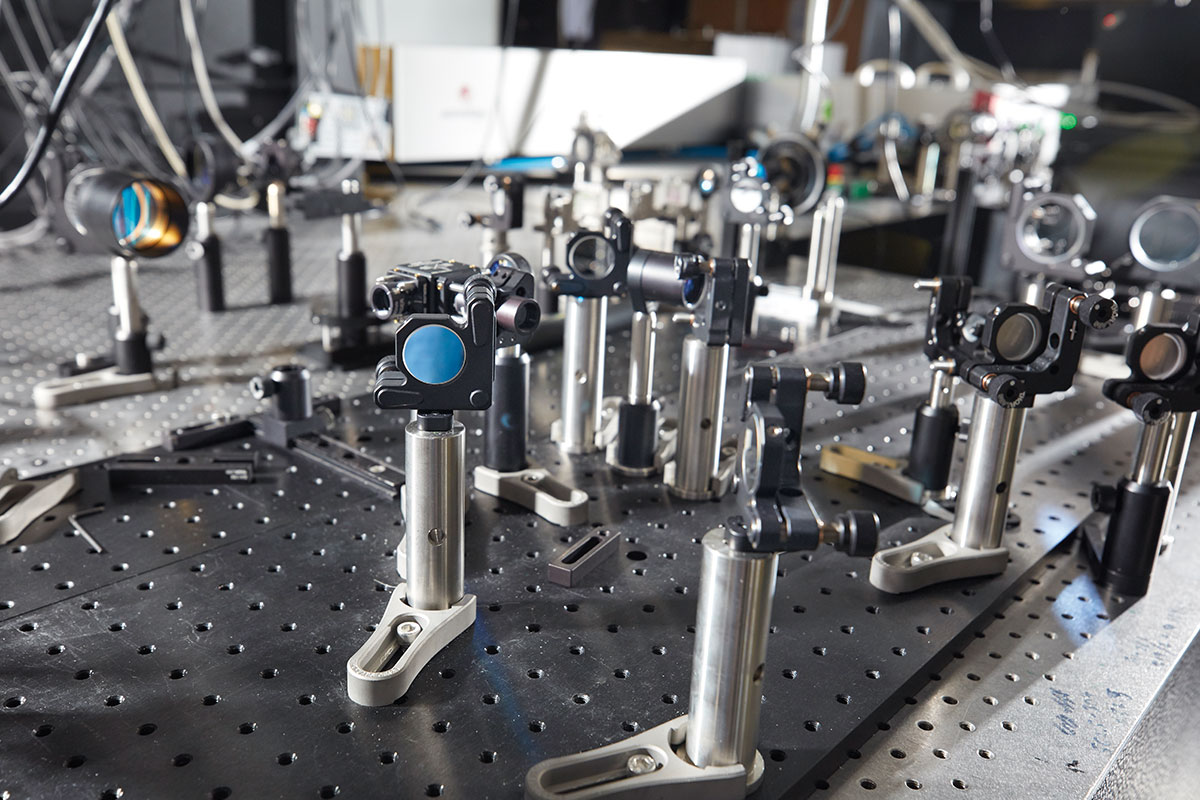
This jumble of cables, lenses, and power supplies may not look like much. But the light-beads microscope is one of the most powerful imaging tools ever invented, capable of capturing activities of neurons within large swaths of the mammalian brain.
“Each neuron can have up to ten thousand connections to other neurons, so superior imaging is crucial if we wish to capture the brain’s structure and function in action,” Vaziri says.
The ideal microscope will be able to visualize neurons with crystal clear resolution as they actively call out from distant corners of the cortex, within a fraction of a second of one another. The fundamental difficulty comes down to resolution, scale, and speed—parameters that tend to be mutually exclusive. Traditional imaging tools often sacrifice scale for resolution, or vice versa. When scientists insist on having both, they typically circumvent the problem by taking snapshots of separate parts of the brain that are later stitched together, a workaround that sacrifices speed and makes it difficult to see action in remote nooks of the brain. And the deeper inside the brain they look, the more challenging the experiment becomes, because dense tissue has a nasty habit of scattering light.
The lab’s latest feat of engineering, light-beads microscopy is so refined that it can record the activity of more than one million neurons across the mouse cortex. It involves breaking one strong photon pulse into 30 smaller subpulses that descend to various depths, each separated by a billionth of a second, making it possible to image very dense tissue. A cavity of mirrors ensures that each pulse reaches its target, causing nearby neurons to fluoresce no matter how deep inside the tissue they are embedded. The method also eliminates the dead time between sequential laser pulses, speeding up the entire process.
Like other imaging techniques developed in Vaziri’s lab, light-beads microscopy is designed to retrieve as much data from the brain as possible. “That’s the spirit of our approach,” he says. “With the minimum number of photons, how can we extract the maximum amount of information?”
The mRNA trap
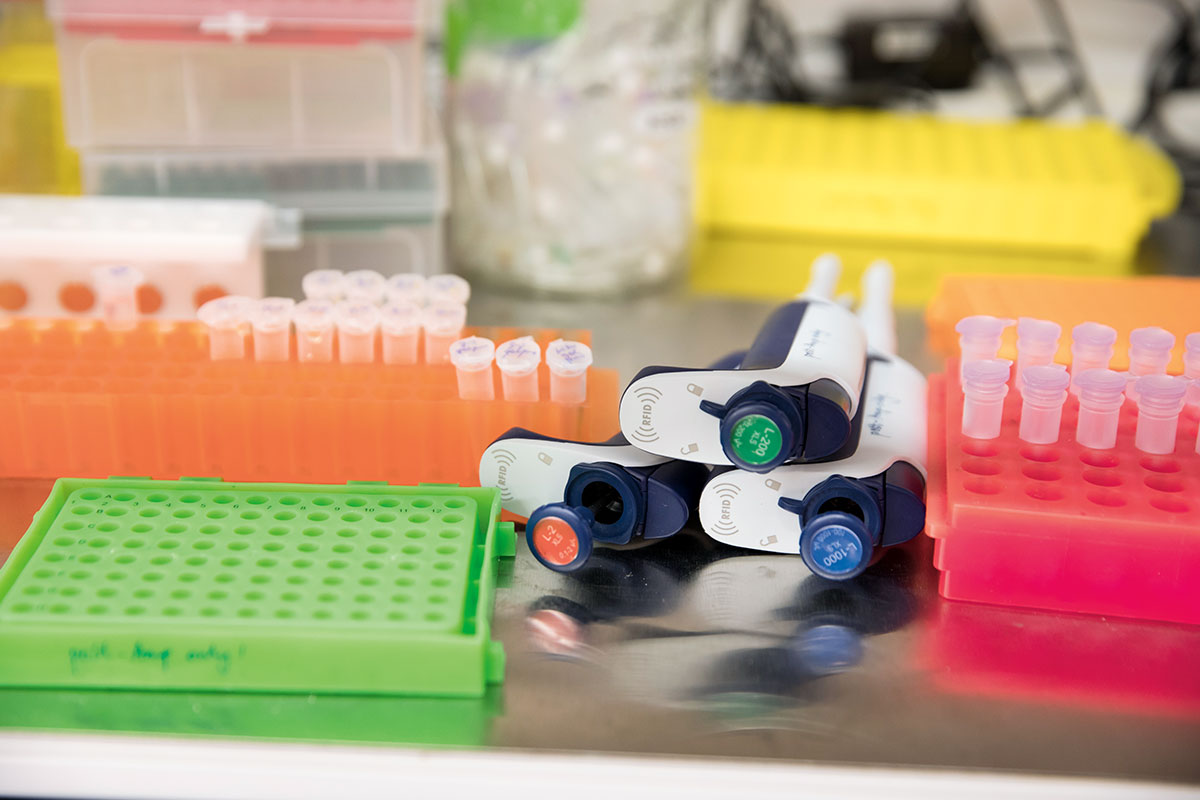
Within ordinary microtubes, a remarkably potent tool is brewing. Dubbed “translating ribosomal affinity purification”—TRAP for short—the method was among the first to allow scientists to identify and sort the hundreds of cell types that make up the human body.
Before TRAP, researchers often studied diseases by isolating a patch of problem tissue, mashing it up, and analyzing all the genes expressed in the hodgepodge of cells. Although this method could identify faulty genes, there was no way to pinpoint the individual cells that produced those genes, so the actual disease culprits remained unknown.
“When you have a collection of 10,000 genes expressed in a tissue sample, figuring out which of those thousands are driving pathology can be very difficult,” Heintz says. “We wanted to know everything happening to the individual cell types in the tissue.”
Along with the late Paul Greengard and then postdoc Myriam Heiman, Heintz realized that they could distinguish one cell type from its neighbor by tracking the repertoire of proteins that each cell type produces—its so-called translational profile. Their TRAP tool literally traps a cell’s mRNAs molecules—the actionable readouts from its genes—as they reach ribosomes, the cellular machinery responsible for turning the RNA sequence into protein. A fluorescent tag makes it possible to extract ribosomes from tissue samples and analyze their associated mRNAs. The result is a unique molecular signature that distinguishes otherwise similar cells from one another.
“TRAP told us everything,” Heintz says.
But optimizing the technique turned out to be a marathon of trial and error. “Decent ideas almost always work in cell culture,” Heintz says. “But making the same idea work in a living organism, or even postmortem brain tissue, can take years.” The team tested different bits of the ribosome to figure out which would be the easiest to tag. And they tested various tags looking for one with strong fluorescence to produce a signal sharp enough to record and profile the cells.
Heintz’s lab now uses TRAP to identify the cells and molecular events driving neurological conditions such as autism spectrum disorders, Parkinson’s disease, addiction, anxiety, and depression. In each case, the team’s approach is to ask how cells differ from one another and what happens to them in disease. “I don’t have any particular favorites,” says Heintz. “I just want to look at as many cell types as possible.”
A harness, built on the fly
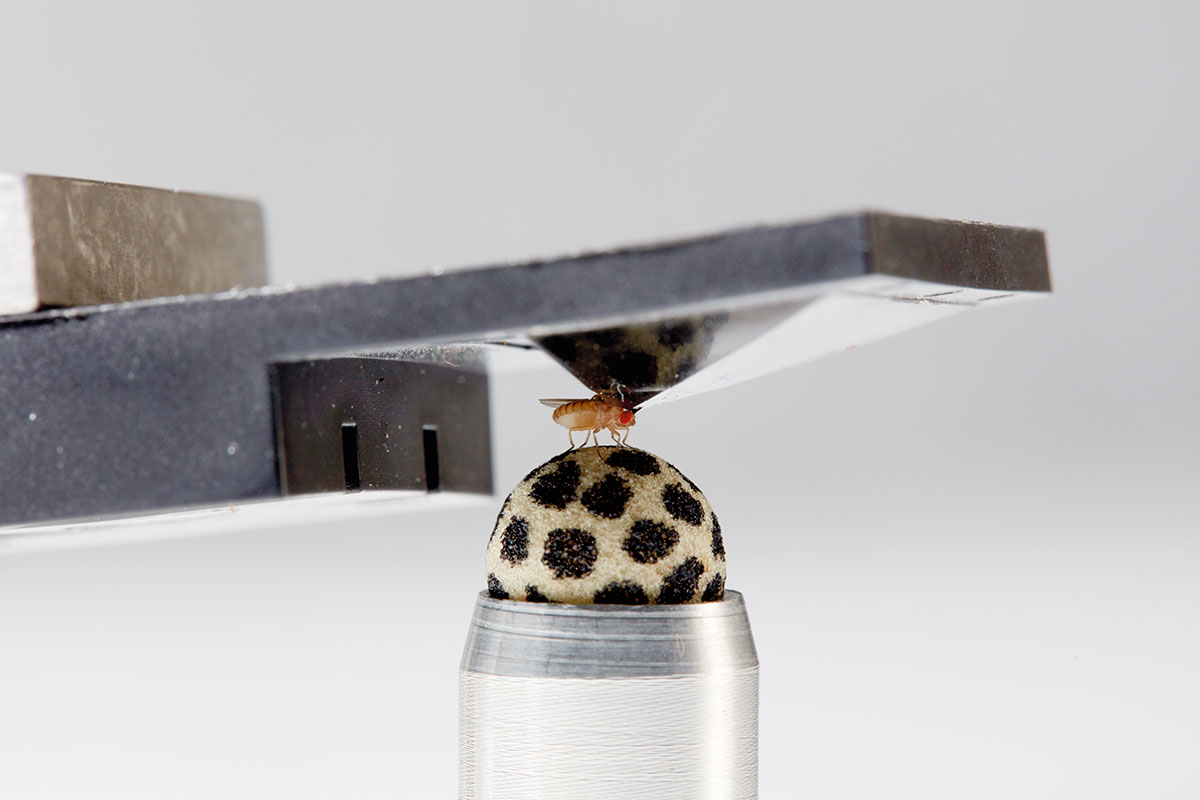
To the uninitiated, it’s a shallow upside-down cup marred by a tiny hole in its base. To behavioral neurophysiologists, it is an invention that transformed the field—a harness that allowed scientists to, for the first time, reliably observe activity in the brains of living, behaving fruit flies.
It was once impossible to study the neurophysiology of fruit flies without immobilizing them. In 2007, when Gaby Maimon was a postdoc at the California Institute of Technology, the method of choice involved cutting a tiny fly-shaped hole in aluminum foil, stuffing the fly into it, and adhering the insect in place with wax. Scientists would then remove a piece of cuticle over the fly’s head and, while bathing the brain in saline, take measurements from neurons as they presented odors to the dry antennae ensconced safely below the saltwater.
But Maimon wanted to study the brains of naturally behaving fruit flies. “The hope was that we could one day make similar recordings, but in a fly that could also flap its wings in tethered flight or perform walking navigation on a tiny, fly-sized treadmill,” he says.
In the laboratory of Michael Dickinson, Maimon began a long process of trial and error to improve on the crude foil harness. If it fit just right, Maimon imagined that scientists would still be able to keep the brain in a saline bath while the animal went (or flew) about its business.
“We contacted several microfabrication companies to make this harness for us, and they asked us to commit to making ten or twenty thousand,” Maimon says. “But mass fabrication made little sense, because we didn’t know if our designs would even work.” So, with help from postdoctoral colleague Wyatt Korff, Maimon set out to make the harness himself on a milling machine.
He churned out one prototype after another. A pyramid-shaped cup too narrow to allow the experimenter to dissect the cuticle. Another pyramid too shallow to allow the fly to flap its wings without hitting plastic. And then, at long last, an efficient harness that allowed the insects to perform tethered walking or flight, as researchers measured signals in the brain.
In this new design, the fly’s head and the front tip of its body were the only parts glued to the “harness” (a hole at the base of the pyramid). The rest of the body remained free, allowing the animal to flap its wings or walk. With this model, Maimon and colleagues have made great strides in their behavioral neurophysiology investigations, including recent work describing how parts of the fly’s brain allow it to calculate its direction of travel or guide it toward a distant goal.
“Even halfway through developing this approach, it wasn’t clear whether it would work, or work reliably, which made life a bit stressful as a postdoc,” Maimon says. But when it finally came together, the novel harness launched a new way to study brains and behavior.
Software for supersized data
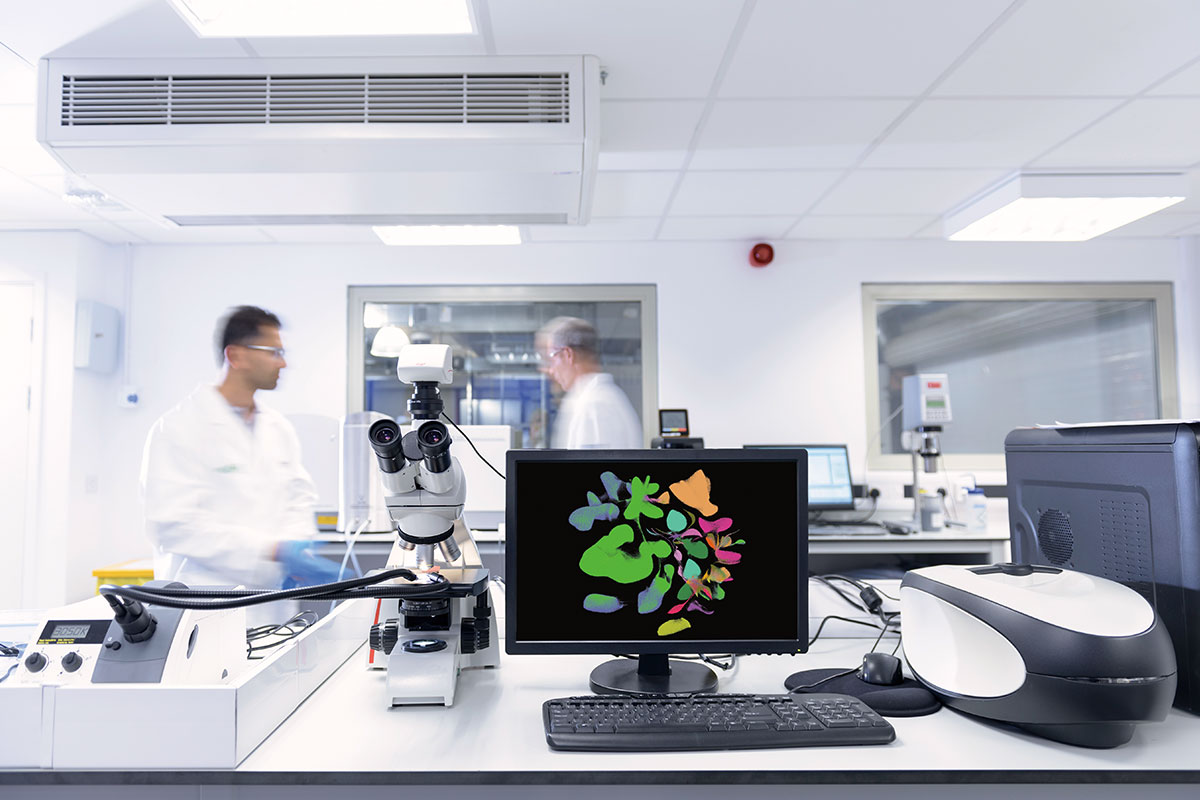
You are looking at one of the most powerful artificial intelligence programs in the world, software tweaked by Junyue Cao into a scientific tool that analyzes enormous biological data sets. It’s innovation by adaptation—a repurposed tool, drawn into the lab from nonacademic sources.
Cao began working with artificial intelligence when the sheer size of his data sets became unmanageable. An expert on single-cell genomics, he and his coworkers are sequencing the genomes of millions of individual cells and analyzing subtle links between them, seeking to piece together a biological puzzle that may provide insights into many human diseases.
“Single-cell genomics faces two key challenges,” Cao says. “One is to visualize huge numbers of diverse cells in low-dimensional space; the other is to identify the gene regulatory networks that operate within multiple layers of cells.”
Cao recently published gene-expression information for four million individual cells in more than 100 types of fetal tissue—the largest single-cell genomic data set to date, which the lab is now scouring to identify cellular interactions that may herald disease. Cao stores the data and runs computations using the high-performance computing systems on campus. “If we used your laptop, it would take weeks to process this data,” he says.
Cao and his colleagues tested and compared many machine-learning algorithms to visualize and classify the main cell types represented in large-scale data sets comprising thousands of genes and millions of cells. Many algorithms worked for normal data sets with hundreds or thousands of cells, but whenever the experiment was scaled up to the millions, the software came crashing down. And even when the researchers arrived at a clustering technique that worked, the program kept making mistakes, detecting links between cells that turned out to have low correlation.
“We again tried many approaches, until we landed on a machine-learning approach that let us manually remove weak links,” Cao says. Apparently, there are some biological questions that AI isn’t ready to answer without at least a little human supervision.
Cao is not a software engineer, and he does not develop the machine learning algorithms from scratch. But he’s learned enough to adapt existing tools to his own needs. The machine-learning approach that his lab finally settled on was based on a technique that social media companies use to distill and track communities among users.
“It turns out, tracking millions of cell interactions is not entirely different from tracking millions of ‘likes’ on Facebook,” Cao says.